
[ad_1]
Researchers from the Indian Institute of Technology (IIT) Guwahati have created the learning-based framework ‘OsteoHRNet’ to evaluate the severity of knee osteoarthritis (OA) using X-ray images. Under the collaborative supervision of Arijit Sur and Palash Ghosh of the institute’s computer science and engineering, and mathematics departments, respectively, Rohit Kumar Jain, an MTech (data science) graduate, built the AI-based model. Prasen Kumar Sharma and Sibaji Gaj, who were both Sur’s PhD students, are also members of the study team. Medical professionals can utilise the model remotely to diagnose the condition more precisely.
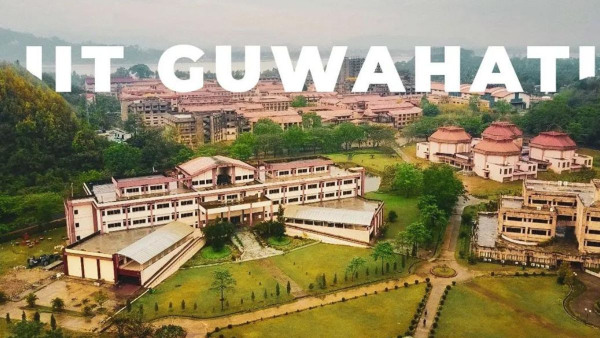
Statement from IIT Guwahati
According to a statement from IIT Guwahati, knee osteoarthritis has a prevalence of 28% in the nation and is the most prevalent musculoskeletal illness worldwide. An early diagnosis is crucial for pain management and behavioural changes because there is no known treatment for knee OA other than complete joint replacement at an advanced stage, it noted.
The statement stated that X-ray imaging is very effective and more economically practical for routine diagnosis. It claimed that MRI and CT scans give a 3D view of the knee joints for effective diagnosis of knee OA but that their availability is restricted and expensive.
According to Ghosh, “Compared to other techniques, our model can pinpoint the area, which is medically most important to decide the severity level of knee OA thus helping medical practitioners to accurately detect the disease at an early stage.”
AI-based model
An effective deep convolutional neural network (CNN), or method from image recognition, is used in the AI-based model. According to the Kellgren and Lawrence (KL) grading system accepted by the World Health Organisation, this model forecasts the severity of knee OA. To capture the multiscale properties of knee X-rays, it is based on one of the newest deep models, the high-resolution network (HRNet).
The proposed approach, however straightforward, might serve as a useful place to start for studying less expensive radiographic modalities like X-rays, according to Sur. At the moment, our research is concentrating on how effectively deep learning-based models can be created so that we can operate with cheap and easily accessible modalities like very low-resolution radiographic pictures or even photos taken from radiographic plates by a smartphone. The team is still working on adapting these models so that they may be used in devices with limited resources.
[ad_2]
Source link